Deep learning: administer the vaccine before the infection takes hold
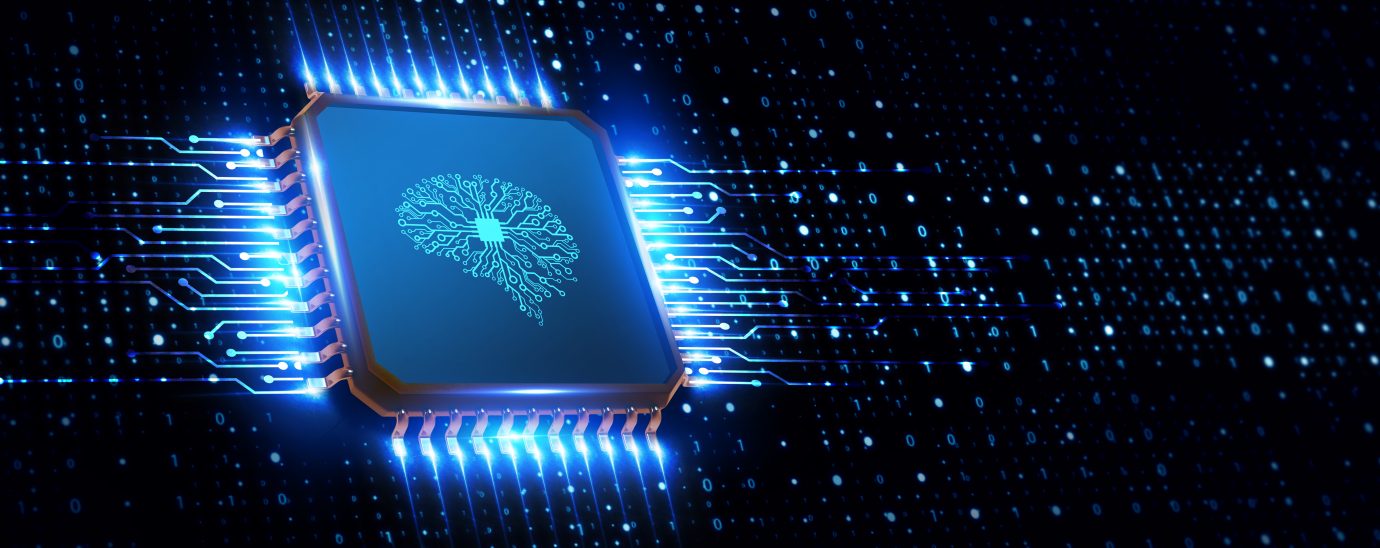
Staying at the bleeding edge of innovation is the only way to outpace attackers. However, most organizations are settling for a mitigation approach to cybersecurity which only engages once the adversaries have breached the perimeter. Attacks then need to run before they’re picked up and checked to see if they’re malicious, sometimes taking as long as 60 seconds. When dealing with an unknown threat, 60 seconds is too long to wait for analysis. A prevention approach is far more effective, explains Brooks Wallace, VP EMEA at Deep Instinct, as it operates on the basis that the attackers are not permitted to come within arm’s length of the network perimeter. And at the centre of this approach is deep learning.
Cybersecurity teams are under more pressure now than ever before. The recovery period after an attack can be a long and painful experience for any business, so teams are naturally looking to increase their efforts to limit the damage caused.
Mitigation has been the name of the game for the past few decades, but organizations realize that the real goal is to stop criminals before they reach the perimeter. Mitigating the impact of a breach is a critical part of long-term security strategies, as there is now a global recognition across the security industry that a cyberattack is a matter of ‘when’ not ‘if’. However, security teams have become overloaded with responsibilities tied to various solutions across the network, to the point that mitigation activity can become counterproductive.
To make matters worse, the market is full of technologies labelled as the next best thing in terms of prevention. With buzzwords like artificial intelligence (AI) and machine learning (ML) being flippantly used for most new solutions, it’s hard to tell which products will provide the required level of prevention to stand against the onslaught of cyberattacks.
Businesses have outgrown past defences
The cyber landscape has changed at such a fast pace that most technologies implemented a decade ago will no longer be up to the job. Priorities over the years have shifted multiple times depending on the latest attack vectors. Many businesses jumped aboard the endpoint detection and response (EDR) train in an attempt to protect themselves from the next breach. But attacks were evolving at such a rapid pace that it was impossible to keep up – especially as at that point, they were already on the backfoot.
Swapping out old technology for the latest developments is the ideal scenario. However, due to budget limitations, this isn’t always an option. Larger companies with deeper pockets can deploy a mature security stack with multiple layers of defence and can therefore be more proactive in their approach to security. Smaller organizations, however, are often forced to take a reactive approach, which is where the trouble begins.
Ideally, businesses need one encompassing solution to add to the security stack that blocks criminal advances before reaching the network perimeter. And luckily, this technology already exists.
The transition from machine learning to deep learning
There has been hype around machine learning over the past few years. It has been instrumental in developing new and exciting technologies that have played an important role in cyber evolution. However, machine learning still has drawbacks that can work against business priorities. As well as requiring teams to regularly input pre-classified datasets to keep the process up-to-date, machine learning has also featured in criminal campaigns, where adversaries use a business’ own machine learning solutions against them. These pre-classified datasets are vulnerable to compromise, and teams could end up inadvertently submitting tampered information into the machine learning system, meaning the programme starts recognizing malicious code as benign, and vice versa.
However, deep learning provides a solution to these vulnerabilities. Deep learning is an advanced subset of AI and has been developed to operate similarly to the human brain. The technology consists of neurological networks, meaning over time, the system can ‘learn’ to recognize and predict known and unknown attacks before they take place. While this technology does require vast amounts of raw data, once the initial set-up process has finished, the system can be left to run with minimal interference from the security team. Fully trained, a deep learning solution can identify and block malware in less than 20 milliseconds.
Deep learning’s capabilities often sound too good to be true, and only in recent years has the technology become viable. Advances in technology such as Graphics Processing Units (GPU) have made true deep learning far more achievable. The rise in awareness is similar to Netflix and Spotify, which 10 years ago were unrecognizable. Now though, they are two of the most well-known brands globally thanks, in part, to their use of deep learning.
Needless to say, prediction and prevention sit at the heart of deep learning. As deep learning only uses raw data, there is no risk of the datasets being tampered with before they’re fed into the system. Therefore, it is far more resistant to adversarial attacks.
One of the biggest issues with having multiple solutions for mitigation purposes is that teams are often inundated with alerts. Imagine 10 different systems sending thousands of alerts a day, each one needing to be reviewed by the security team to evaluate the level of risk. Enter deep learning.
READ MORE:
- Twitter to use deep learning to crack down on fake news
- Robot rock: how deep learning is central to Spotify’s strategy
- How the deep learning skills shortage is impacting businesses
- European IT leaders say flash-based object storage will fuel AI and ML
Once integrated into the existing security stack, deep learning intuitively calculates any potential risks and only alerts the team when further review is needed. Not only does this process contribute to the prevention strategy, but it also reduces the number of alerts by a minimum of 25% each week. Using raw data, deep learning will intuitively predict potential threats and vulnerabilities and will only alert the team when a genuine risk is identified. Everyone can continue with their day in full confidence that the deep learning system is in control, knowing that the technology can instantly identify malware.
The shift to prevention
Let’s compare the scenario to a physical office. We would much rather have a solution that identifies and blocks burglars before they breach the building, rather than one that prides itself on being the quickest to boot the bad guys out once they’ve broken in. No matter how fast the detection and response systems are, the initial damage has already been done. And given that some malware can take hold only 15 seconds after entering the system, most defences will engage too late.
The average cost of a data breach now stands at a shocking US$4.24mn. The time for mitigation has come to an end, and teams must hold prevention at the heart of all future security developments. Even allowing attackers inside the perimeter for a matter of seconds is too long. While detection and response systems have played an integral part over the years, they no longer stand strong against today’s advancing threats. Deep learning holds the key to attack prediction, and can help lead the charge into a secure future based on prevention over mitigation.
For more news from Top Business Tech, don’t forget to subscribe to our daily bulletin!