Crowds deliver diverse data sets to produce effective AI
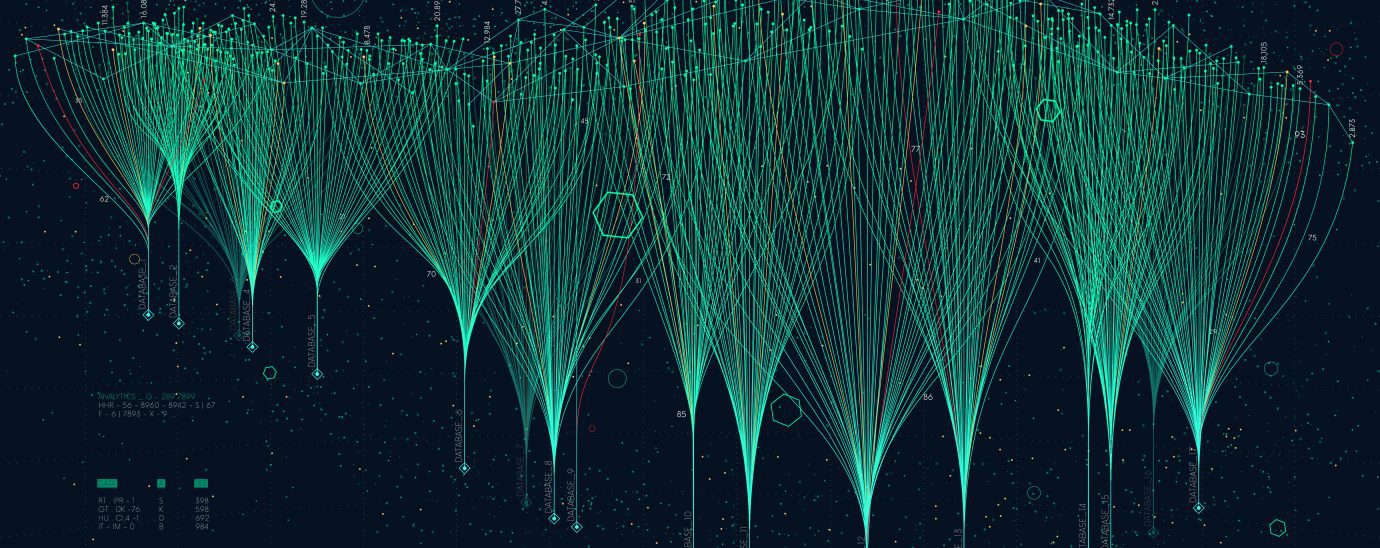
Deriving the greatest benefits from AI requires businesses to understand and be comfortable with what it can and can’t do, and how it should be leveraged for the best results. In this article, Jonathan Zaleski, Sr. Director of Engineering at Applause and Head of Applause Labs, explores how businesses can overcome any difficulties associated with implementing AI and automated systems, and the importance of successfully training and crowdtesting their AI/ML models to remove any potential harmful bias.
Defining purpose and overcoming objections
Before deploying AI, businesses should always begin by clearly defining the need for the technology in their organization, asking themselves what purpose it will serve and how it can be used to accomplish that original objective. It’s important, too, to establish where AI isn’t needed. Many organizations, for example, don’t understand that not all their business processes can – or need to be – automated. Only once its purpose has been defined can AI begin to be used for best effect.
Any AI deployment is likely to face some resistance, of course. The age-old concern that AI represents a threat to people’s jobs can often be overcome by demonstrating the efficiency benefits that automation offers when compared to time-consuming, traditionally manual tasks. Addressing the issue of bias in AI, however, can be a little more challenging.
The issue of bias
An AI’s basic purpose and functionality are fed into its underlying algorithm. But, if the AI was to develop an inherent bias, it would have a detrimental effect on that algorithm, which could seriously impact the precision and efficiencies the AI is expected to deliver. This, in turn, can limit its ability to fulfil its commercial requirements, and that can be bad for business.
Unfortunately, despite the best intentions of developers, bias can always find a way to permeate an AI algorithm. Biases based on business decisions, training data, and even conscious bias, continue to crop up. And, as well as affecting efficiency, such bias can also negatively impact the perception of a brand. Unintended gender bias, for example, resulted in the Apple Card offering lower credit limits to female applicants than male. The resulting backlash on social media was, unsurprisingly, harsh. If a customer feels they’re being treated unfairly by an AI system, they’ll think twice about engaging with that particular brand again.
Examples like this only add to the skepticism around AI and can make it difficult for businesses to justify investing in the technology. To avoid such situations occurring in the first place, businesses should therefore place more emphasis on the training of their AI algorithms and consider a crowdtesting approach to ensure a suitably diverse data set.
Real-world training and testing
Every successful AI algorithm is built on training data. But, with AI, as with any learning process, the student is influenced by the teacher. The scope of an AI’s education is dependent on the curriculum. So, it stands to reason that a more varied and diverse curriculum will produce a more enlightened student. Likewise, using a larger and more diverse data set will help to produce more precise and efficient AI algorithms capable of making smarter decisions, and with less inherent bias.
Sourcing the data needed to meet a business’s requirements can be challenging, though, especially for mass market consumer applications and services. In-house teams of developers, software engineers, and quality assurance specialists will typically be from the same age range, gender, and socio-economic background. As a result, bias can often occur during the process of collecting and labelling data. It’s best, then, when building an AI algorithm, not to rely on a single person or small group to provide the data that’s going to be used to train that algorithm. To properly train it, and minimize the risk of bias, requires different types of data and inputs.
It would be far more productive to use crowdtesting, a model that provides the AI algorithm with exposure to a diverse pool of people and experiences which are much closer to the customers it’s designed to serve. By using this model, businesses can train their algorithms to respond to real-world scenarios, detect where biases occur, and reduce their potential impact.
Rich variety of data and inputs
An AI algorithm needs to be tested under real-world conditions, interacting with real people that mimic a company’s target audience to ensure it works as intended.
Businesses need to source training data from a pool that provides quality and diversity – as well as quantity. Indeed, without diversity in the training data, the algorithm won’t be able to recognize an especially broad range of possibilities, thereby limiting its effectiveness. The necessary diversity and scope of data can be found in carefully vetted communities of testers offering specific demographics – including gender, race, age, geography, native language, location, and skill set, among others.
Without exposure to such a rich variety of data and inputs, AI can fail to deliver on its potential, having been limited only to in-house lab testing practices. By supplementing an organization’s in-house capabilities for training algorithms to study and recognize voices, text, images, and biometrics, for instance, this crowdtesting approach can provide businesses with strong outputs that will service the needs of a diverse customer base.
Delivering on its purpose
AI technology represents considerable efficiency benefits for businesses. It’s important to understand, though, how AI will deliver those benefits, and the issues that could hinder its efficiency and its wider acceptance.
Businesses need to appreciate that, while AI will never be perfect, it’s constantly learning, and the best machine models are those based on large and diverse data sets. Without diversity in the training data, the AI algorithm will be unable to recognize a broad range of possibilities, which risks rendering it ineffective. What’s more, inherent biases arising from limited input can impact not only the AI’s efficiency and precision, but also the reputation of the business using that AI.
READ MORE:
- Social justice and sustainability by leveraging data science and AI: Interview with Dr. Mahendra Samarawickrama
- Explainable AI for text summarization of legal documents
- It’s time for AI insurance
- Why it’s a commercial imperative that your team develops AI skills
The best policy, then, is to take a crowdtesting approach, and source that training data from a pool that provides quantity, quality, and diversity. That way, an organization’s AI will be best placed to deliver on the purpose originally defined before its deployment.
For more news from Top Business Tech, don’t forget to subscribe to our daily bulletin!