Overcoming The Biases: A Data Or A People Problem
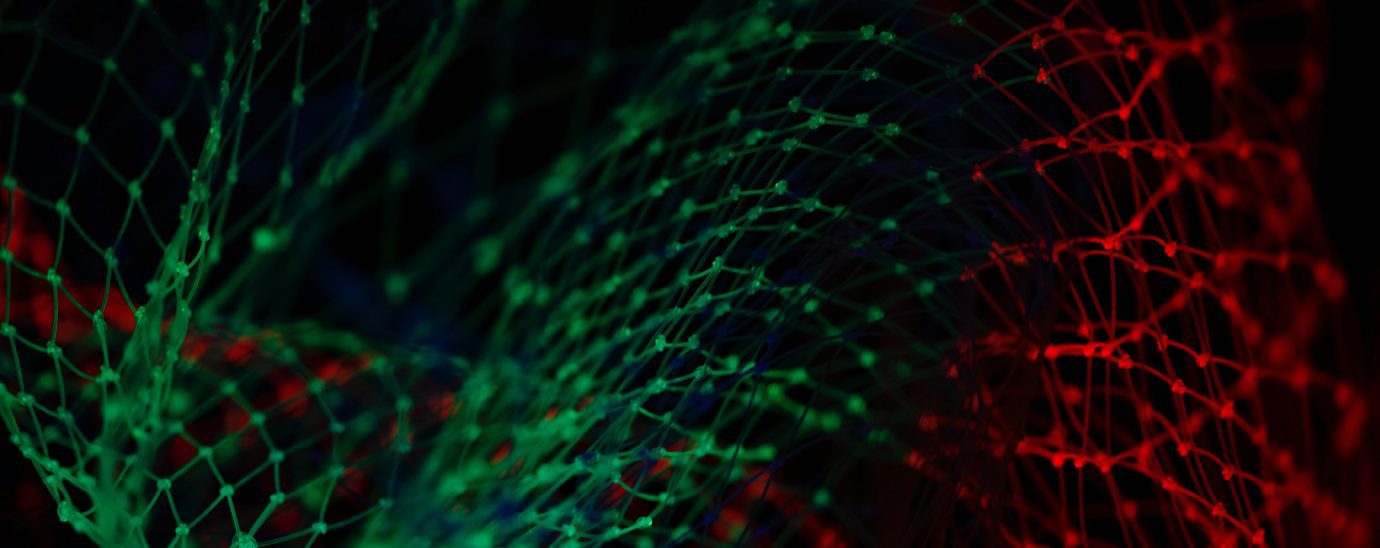
‘Data’ and ‘facts’ are interchangeable terms, right? Unfortunately, not. A real challenge in data can be how it’s interpreted, and the bias that it might hold.
Bias is a people problem, but it can influence data in a big way. Bias is a natural component of the human thought process and as such can be inherently embedded within data and the stories it tells. Data patterns and algorithms are created by people and consequently often mimic human behaviours. For this reason, the fact is almost all big data sets, generated by systems powered by ML/AI-based models, are susceptible to bias.
There are many adverse impacts of bias in data analysis, from making bad decisions that directly affect a company’s bottom line to having the potential to reinforce (or even accelerate) racial, political or gender inequalities, and paint a misleading and distorted picture of the facts on the ground. Although data helps us see the world like never before—becoming aware of and taking preventative measures against data biases is an important step to making better decisions with data.
Avoiding bias starts by recognising that data bias exists, and the key is to be sceptical and question everything: who created the data? Why did they create the data? How was the data created? In his latest article, Simon takes a look at the different types of bias in data analysis and how modellers can address biases before problems arise.
- Types of Biases in Data Analysis
- Root Causes of Data Biases
- The Impact of Biased Data
- Overcoming Bias and How to Make Smarter Data Decisions