AI in Healthcare: Applying the Automation Spectrum to Business
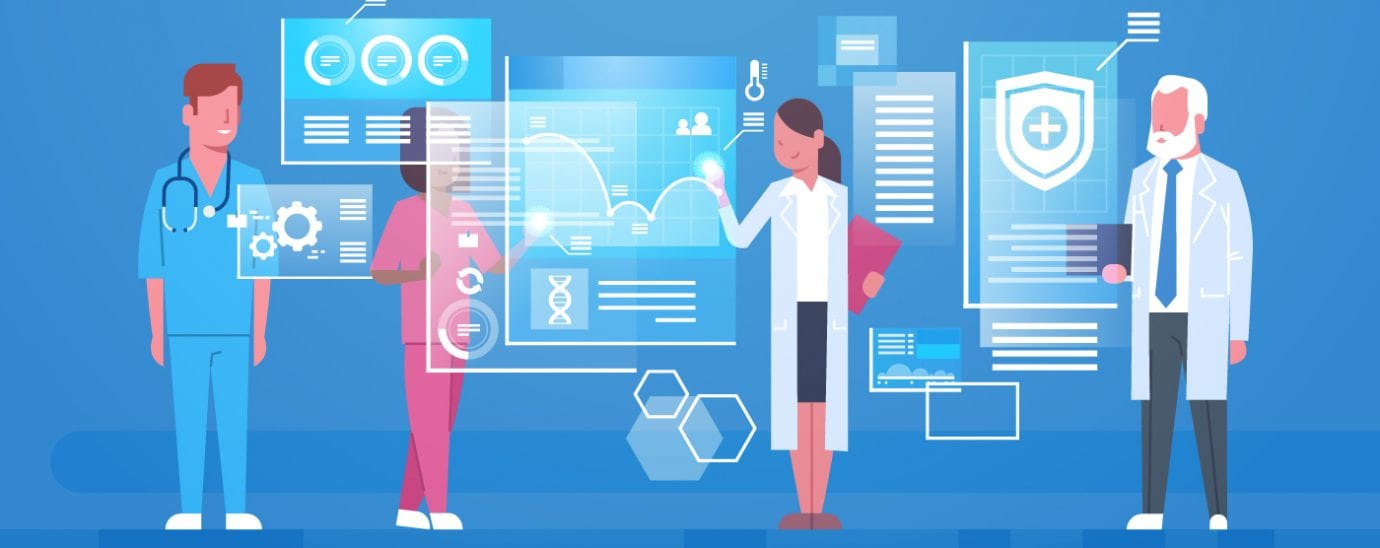
In our latest contribution Vinay Ramani, CPO of Pipedrive, discusses precision within AI in healthcare.
With Google’s AI system recently demonstrating that it can accurately diagnose 26 skin conditions to the same accuracy as US board-certified dermatologists, there is an increasing buzz around the potential of AI in automation. The capabilities demonstrated by this AI were published in a paper titled ‘A Deep Learning System for Differential Diagnosis of Skin Disease’ and was received with wide acclaim. Despite this, the British Skin Foundation, and their American counterpart, list common skin diseases numbering closer to 70. What this means is that Google’s AI system, while impressive, is only capable of detecting 37 per cent of common skin conditions. As such, we may be tempted to ask what is stopping it from detecting all skin conditions?
A good place to start is looking into precision and recall. By precision, we are referring to how accurately a machine can make a prediction for a particular question. Precision is not something that machines can achieve immediately or from the moment they are powered on; it requires significant training through exposure to vast amounts of data. Only through this process can AI begin to approach the levels of accuracy we might expect of a human and this is for only one specific use case. It becomes increasingly challenging to develop AI as the number of use cases they need to satisfy increases. To demonstrate this via example, let’s look at Google’s AI and it’s precision. While it is “on par” with a US board-certified dermatologist, this AI is only valuable in this one specific use case. By contrast, your average dermatologist can likely perform a number of other tasks with great accuracy. To develop an AI that could entirely perform their role, at the moment, is too technologically-challenging. That being said, as the number of use cases increases the recall of an AI increases as it has more experience from which to make predictions and evaluate.
READ MORE: NHS establishes AI lab to improve healthcare
With these two different criteria in mind, we can now begin to look at the ‘automation continuum’. Automation is the use of technology to enable a machine, process, or system to perform activities more quickly, with greater accuracy, and without biases compared to human workers. In basic examples, automation is simply a machine following predetermined rules to complete simple and repeatable tasks. For instance, robots using workflow software to machine, weld, paint, and assemble parts in certain manufacturing environments.
On the other end of the spectrum where we have more advanced examples, automation can enable machines to simulate human behaviour in a variety of ways. Often in these more advanced use cases, the automation can improve as the underlying AI learns and adapts without the necessity for human intervention. We can see this in cashiering at fast-food restaurants and automated tellers helping a customer open a bank account. That being said, even more advanced examples are now also capable of performing less predictable or higher-skilled tasks that augment and extend human capabilities. This allows for routine administrative tasks to be offloaded from workers and free up their time for value-on activities. This is going to play a pivotal role in industries such as accountancy, law or finance where repetitive and time-consuming tasks are a hindrance to other activities.
For these more advanced use cases, artificial intelligence is a key driver. AI and machine learning are the technologies underpinning these capabilities and performing tasks once considered exclusive to human beings. This is due to the fact that AI has the capacity to understand and interact using human speech, interpret and match images, recognise patterns in data, make recommendations, predict outcomes, and independently learn from experience. Moreover, by combining information from a variety of inputs these AI-based systems can self-adjust based on previous successes and failures.
In summary, deciding which end of the automation spectrum is the most suitable for your business use-case depends on two factors. Firstly, what inputs you have available to input into the AI and, secondly, what your needed output is. On the basic end, where the desired output is simple and there are restricted inputs – basic automation is the best choice. For more complex outputs, however, strong-AI integrations are necessary. As such, if you’re thinking about introducing automation or AI into the workplace, make sure you carefully consider what’s going to achieve the best outcome for your business.