Supply chain automation: Maximising the value of IoT data
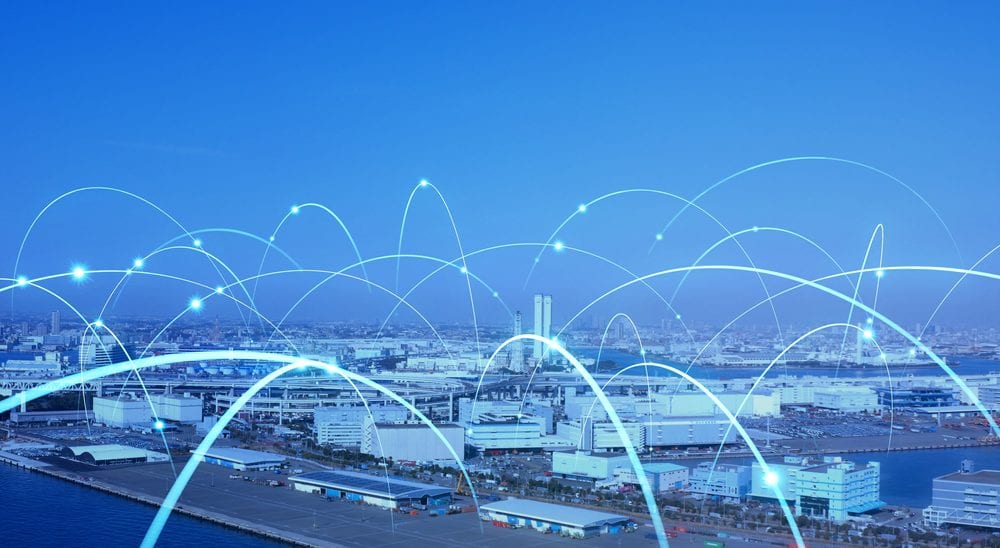
Christophe Vaissade, Sales Director EMEA at Western Digital shares a few simple steps to help enable businesses to maximise on their IoT data for supply chain automation.
As world trade becomes increasingly more complex, today’s supply chains are evolving to reflect this highly competitive and increasingly volatile environment. In a world where customer requirements can change in an instant, a one-size-fits-all supply chain model can exacerbate inefficiencies, and add unnecessary complexity.
To break free from standardised supply chain models, it’s time for companies to make the most of automation. Many businesses are sitting on a wealth of historical supply chain data that, if utilised correctly, has the potential to power a fully-automated and efficient supply chain. It doesn’t come as a surprise that data analytics is the next big thing in supply chain management, with business leaders ranking advanced analytics as the second most important emerging technology.
There is huge value in running analytics on data that is captured at the edge, but there are also use cases that make sense for storing some of this data locally. In addition to capturing this data as primary or backup storage, edge storage and compute devices will maximise network efficiency and enable systems to analyse the data and act on the results in real-time.
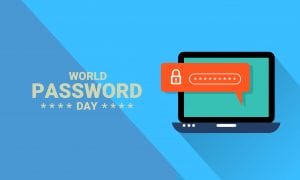
However, it isn’t always obvious how this can be put into action. By following a few simple steps, businesses can use machine learning and advanced analytics techniques to design a fully-automated prediction model, creating much more efficient and customer-centric supply chain processes.
Design an optimisation framework
When developing a supply chain optimisation strategy, it’s important to establish a comprehensive understanding of the business objectives, the organisation and its needs. The framework should consist of four pillars:
- Foundation – establish connected enterprise systems plus big data infrastructure and data governance, in order to support the new model
- Network landscape – streamline the carbon footprint, rationalise the carrier base and optimise the network model
- Team organisation – create a control tower to help with change management, develop talent and focus on culture
- Digital solutions – implement intelligent transportation solutions, smart warehouses and utilise emerging technologies where appropriate
It’s important to take a balanced, bi-modal approach when putting this framework into place, rather than being too flexible or too rigid. For example, it’s beneficial to use disruptive, highly adaptive methods that ensure failures happen quickly, thereby speeding up the learning process. As well as this, it helps to continuously identify and act on opportunities to improve these processes as you go along. Together, these methods of ‘continuous innovation’ and ‘continuous improvement’ will enable the business to mature its technology quickly, efficiently and cost-effectively.
Manage data collection throughout the ecosystem
Some companies deliver tens of thousands of unit shipments every week to customers, channel partners and more – meaning there’s a lot of data to be processed. It’s important for organisations to manage a data strategy throughout the edge-to-core ecosystem, addressing the unique challenges in these environments. There are a few considerations to ensure the data can be successfully captured at the edge in these environments:
- Data collection at the edge means sensors and storage are not residing in a quality data centre, but are out in the elements. That could mean collecting data for days or weeks in extreme high or low temperatures or while aboard moving platforms where they must be able to withstand unpredictable vibrations. Edge environments can be significantly harsher than traditional mobile, client or data centre environments.
- For many large businesses, a data lake or centralised data warehouse is the only solution that is capable of handling such large volumes of data, and should be a single-source of truth. In order to build this warehouse, data should be ingested from integrated systems, transportation management through to workload management and freight forwarders.
- Once the warehouse has been built, it can be used to feed directly into the data model and analytics platform. From here, the platform can be used to derive insights such as the most efficient shipping routes, and the best days to ship. Adjustments can be made within the transportation management system to improve reliability, speed up transit time for inventory, and increase shipment consolidation.
Develop forward-looking models
By adopting a fail-fast approach, drawing insights from the data and working with colleagues from other departments, businesses can move from simply managing and connecting supply chain operations to using predictive analytics with machine learning. This will ultimately make the supply chain much more adaptable to changing customer requirements.
Moving forward, there is potential to develop and deploy forward-looking models, which will allow businesses to compare different scenarios, understand potential outcomes, and push the most effective alternative into production. Ultimately, this will enable businesses to drive transit times, routes, and shipping schedules down to the delivery-address level, thereby establishing a much more reliable and efficient supply chain.
There are many exciting opportunities across industries for maximising the value of IoT data, whether that be capturing and processing data in real-time at the edge, or applying machine learning to larger data sets at the core. Effective data storage and data analytics is the key to this as it will enable organisations to harness rich historical data to guide strategy as well as using data gathered from IoT-enabled devices to collect supply chain data about shipments in-transit.