Keeping AI cost effective in the move to cloud
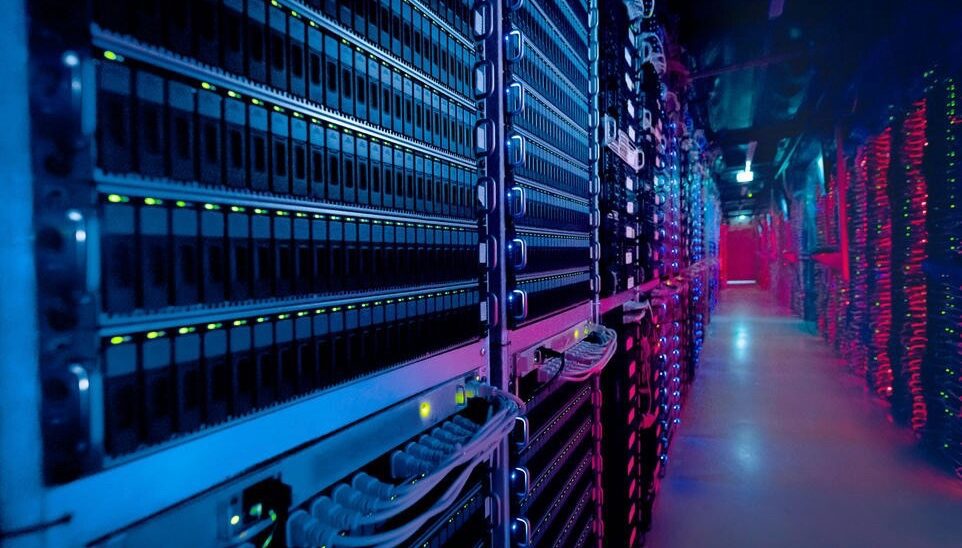
Can Artificial Intelligence Deliver Real Value Today?
AI is in its infancy, but the early shoots of growth hold great promise for the industry. According to the Boston Consulting Group, although 59% of organisations have developed an AI strategy, and 57% have carried out pilot projects in the area, only 11% have seen any kind of return from AI. That said, the potential is vast; some sources estimate that the size of the global AI market could increase tenfold from $15bn (2021) to $150bn by 2028, and in the UK, expenditure on AI technologies could reach £83bn by 2040, from £16.7bn in 2020.
Whatever the application, most AI projects usually start as small, experimental tests hosted on a server in-house, and eventually graduate to cloud environments, where their uptime, security, scalability and maintenance can be assured. However, this migration – the ‘teenage’ stage of an AI application’s lifecycle, as it were – is often the most difficult and painful.
Growing Pains
Moving an AI application to the cloud isn’t just a matter of ensuring greater scalability and improving uptime – it’s often a matter of cost. AI applications usually rely heavily on GPU and GPU-like processors, which can be a significant investment for any startup or lab. Although a single specialized card can be found at around a thousand pounds, more advanced, high-performance GPUs can be in the region of £5,000 to £15,000 each. Delivering this level of high performance at scale is often out of the question from a CapEx point of view, especially for a start-up.
Furthermore, AI application developers eventually reach the limits of their in-house machines; AI usually needs to be trained on exceptionally large datasets, which can mean running out of RAM and storage space fairly rapidly. Upgrading to a high-performance machine in the cloud can remove this bottleneck at both the development and production stages. However, there are a number of things that teams should be aware of and prepare for if they are to make the migration to cloud as painless and productive as possible.
When Plans Come Together
In the very early stages, research and preparation are key. For example, portability is key; working on a platform like Docker from the get-go can greatly help you before and after migration. Even before moving to a third-party datacentre, working in a containerized environment means that your coworkers and collaborators can quickly replicate the app and its dependencies and run it under exactly the same conditions as you have, allowing for robust and reliable testing. However, having an AI application running in a container also means that you’ll minimize re-configuration during the migration process as well.
From a provider point of view, it’s worthwhile understanding the credentials of cloud companies; for example, is their security regularly audited by independent bodies? Do they have specific security accreditation from the vendors they use in turn? AI applications can often handle extremely sensitive data – from simple chatbots in retail banking, to complex healthcare analytics systems, for example – so making sure that this data will be handled, stored and protected appropriately is a must.
Similarly, sustainability is an important consideration. AI requires high computing power and the Wall Street Journal recently revealed that handling a search query via ChatGPT was seven times more compute intensive than a standard search engine. In fact, the University of Massachusetts Amherst research team found out that the GPT-2 algorithm (ChatGPT’s older sibling) created approximately 282 tons of equivalent CO2 – a similar amount to what the entire global clothing industry generated in producing polyester in 2015. AI application developers should be considering sustainability from the get-go, as well as how their partners manage recycling and electronic waste.
At a more specific level, it’s also important to be clear about scaling. Having clear discussions with cloud providers about the specifics of app functionality, who will be using the app, and what that means for the technical architecture, can make sure that no aspect is left neglected. After all, most large-scale cloud providers can offer automatic and unlimited scaling, but there’s a lot of difference between the set-up needed for a system getting ten requests a day and one that gets ten thousand in a minute, so it’s important to be clear about instance ranges, for example.
Similarly, latency considerations are crucial; the likes of chatbots and other real-time systems need to respond instantly to web users. Consequently, this means that both code and infrastructure must be sufficiently low-latency, and developers and deployers will need to shave off every possible milli-second. In terms of deployment, this means checking that compute resources, for example, are as close to (or in the same place as) data, which will help to keep things as fast as possible.
Finally, once the application has been deployed, continuous monitoring is important. There may be alternative configurations within the cloud provider’s environment that could better suit its needs – or in some cases, moving to an alternative provider may be the best thing for the app. Working with open standards, in an open-source cloud environment such as OpenStack, can often make this less challenging.
When AI Grows Up
Nobody knows if AI will ever reach the lofty – and sometimes terrifying – heights that science fiction films have promised for decades. However, if this incredibly promising and powerful technology is to reach its full potential, especially in the face of the current energy crisis, it needs to be deployed as efficiently and effectively as possible and allow its creators to focus on their core work, building AI systems, rather than worrying about infrastructure and operational concerns.
If AI developers can plan carefully, choose their partners well, and streamline their processes when they move applications from their on-premise training-wheels environment to the bigger, wider and more flexible world of cloud, then they will considerably increase their chances of successful re-deployments, keeping costs down and end-users happy. And although that’s not the same as building WALL-E, a T-1000 or Chappie, it’s a step in the right direction.