How can we debias artificial intelligence?
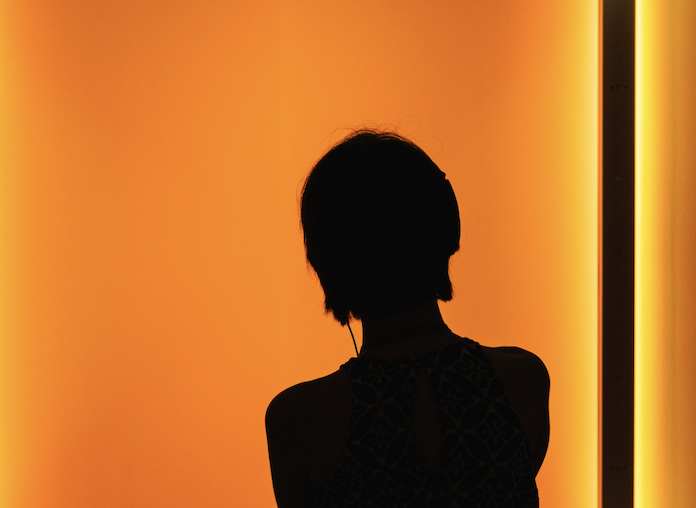
Artificial Intelligence is already playing a huge role across the financial services sector, the medical profession, the world of recruitment and many other important areas. However, current AI only works well if the right information is used to train it. But when humans are the ones collecting that information, it opens the door to bias. So how do we ensure debiasing in AI?
Understanding the process
AI is not yet able to think for itself, therefore, human assistance is required in assembling the necessary data it needs. It’s a bit like programming any other computer in the sense we only get good results or correct decisions from the right information and correctly organized. Get this wrong and the results can be devastating.
For example, in training recruitment algorithms based on historical data, we had AI that favored young, white men and exclude women and people of color. In short, the training data here was clearly wrong and biased. Understanding this can help us begin the debiasing process as some models will need vast amounts of data to help them make sense of the world. Something as simple as getting the correct associated metadata linked to demographics is vital.
Asking the all-important questions?
When using AI, legislation (and common sense) dictates that we need to look at how it might have come to a certain decision or series of answers, especially when we aren’t totally happy with the result. To this end, there’s already a growing area of research into ‘explainable AI’, whereby we can look at a set of models and unearth what decisions are based upon.
Ideally, explainability is built into the model itself. But even so-called black-box models can be run in such a way that we can, through a little trickery, infer which part of the input data had the greatest impact on the classification given by the network in question.
This is important as all individuals in Europe now have the lawful right to have automated decisions explained to them, and so transparency is everything. HMRC has been under fire in recent years for a lack of transparency around how they use AI to make decisions on which people should get universal credit for example. This may not infer bias of course, but transparency is certainly the key word here.
Taking steps now
Why do we need to be so concerned about ensuring the process of debiasing gathers pace right now? Because AI has already become a really important tool in our ever-evolving digital world. We need to understand how we can use this concept to get the best results for everyone concerned.
In terms of the sectors utilizing AI, the decisions made can have a huge impact on people’s lives. What we don’t want is to nurture an age of discrimination simply through training in the wrong data.
Medics are increasingly using data-driven algorithms to reach decisions on healthcare via AI: These are informing healthcare decisions such as diagnosing cancers, heart conditions and eye diseases. There are new advanced applications on the way. But there are dangers lurking in training in the wrong data.
Using a recent American example in the field of health care, a widely adopted algorithm clearly discriminated against black individuals by linking up care with costs. The problem here was AI concluded as Caucasians spend much more money on healthcare, black people must be healthier generally. Given the current healthcare system in the United States, this assumption was dangerously wrong of course.
It’s important to note when large-scale personal bias emerges, we can trigger a series of negative reactions. For people getting the right treatment, a job on their real abilities or for those looking for valuable insurance, eradicating bias in our AI approach is priceless. The bottom line is, that the wrong approach can lead to failing businesses, wrecked lives, inflated prices and all sorts of negative scenarios. We need more work carried out on debiasing information at the point of collection.
The conclusions
There’s little doubt bias, whether it be gender or racially based emanates from basic human prejudice. When it comes to using AI in decision-making, we can see that the low quality of data that this human bias generates can produce alarming results. This bias will be amplified resulting model.
We must clearly design all AI models with inclusion in mind, perform targeted testing in complex cases and train in complete and representative data. This will all help ensure the process of debiasing continues to improve.